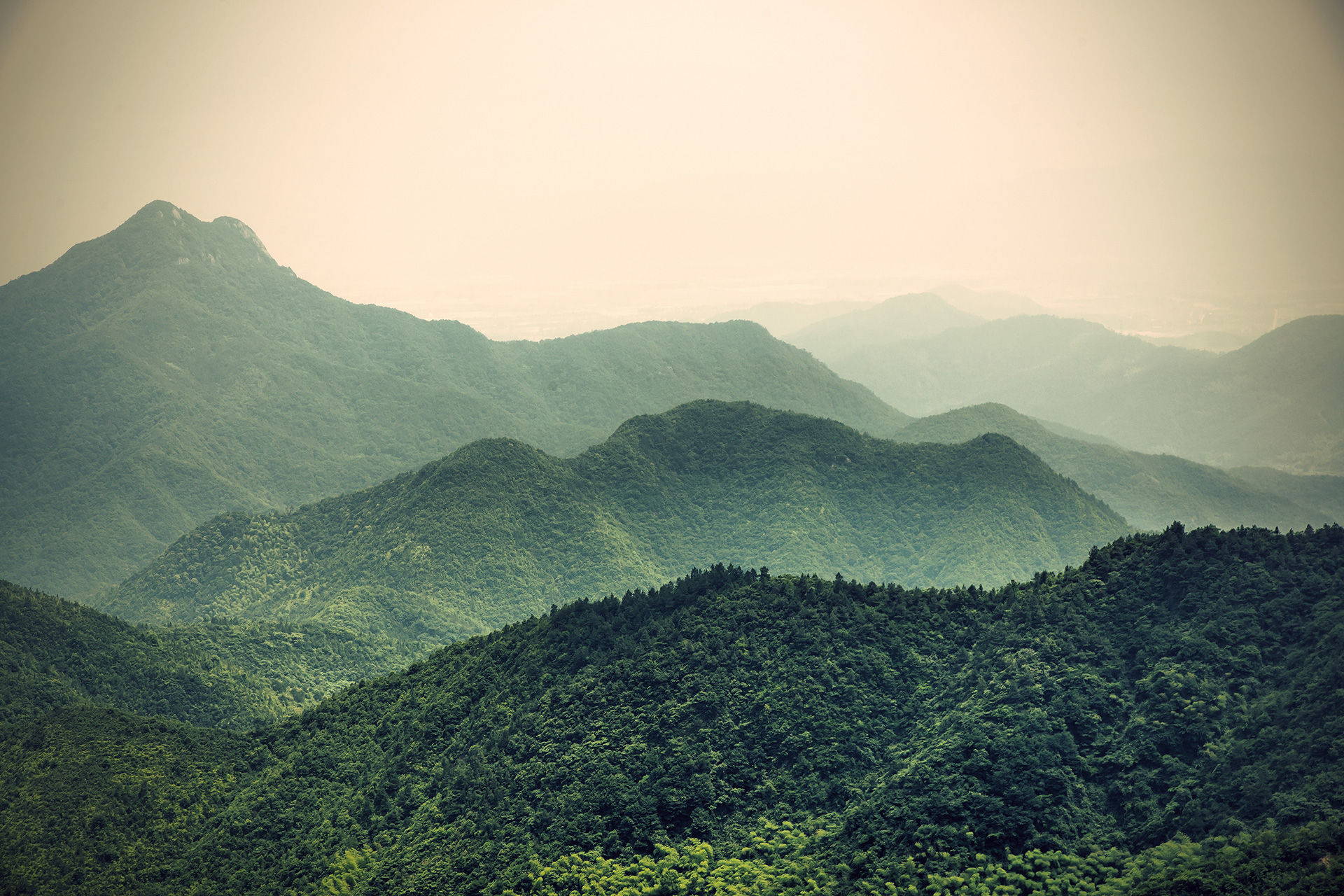

ACCESS
Data Analytics Workshop
KTH Royal Institute of Technology, Stockholm
15 May 2017
Speakers

Prof. Georgios B. Giannakis
ADC Chair in Wireless Telecommunications & McKnight Presidential Chair in ECE, University of Minnesota
Short Bio: Georgios B. Giannakis (Fellow’97) received his Diploma in Electrical Engr. from the Ntl. Tech. Univ. of Athens, Greece, 1981. From 1982 to 1986 he was with the Univ. of Southern California (USC), where he received his MSc. in Electrical Engineering, 1983, MSc. in Mathematics, 1986, and Ph.D. in Electrical Engr., 1986. He was with the University of Virginia from 1987 to 1998, and since 1999 he has been a professor with the Univ. of Minnesota, where he holds a Chair in Wireless Telecommunications, a University of Minnesota McKnight Presidential Chair in ECE, and serves as director of the Digital Technology Center. His general interests span the areas of communications, networking and statistical signal processing – subjects on which he has published more than 400 journal papers, 680 conference papers, 25 book chapters, two edited books and two research monographs (h-index 120). Current research focuses on big data analytics, wireless cognitive radios, network science with applications to social, brain, and power networks with renewables. He is the (co-) inventor of 25 patents issued, and the (co-) recipient of 8 best paper awards from the IEEE Signal Processing (SP) and Communications Societies, including the G. Marconi Prize Paper Award in Wireless Communications. He also received Technical Achievement Awards from the SP Society (2000), from EURASIP (2005), a Young Faculty Teaching Award, the G. W. Taylor Award for Distinguished Research from the University of Minnesota, and the IEEE Fourier Technical Field Award (2015). He is a Fellow of EURASIP, and has served the IEEE in a number of posts including that of a Distinguished Lecturer for the IEEE-SP Society.
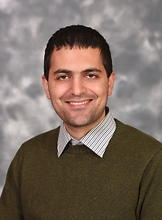
Short Bio: Raja Giryes is an assistant professor in the school of electrical engineering at Tel Aviv University. He received the B.Sc (2007), M.Sc. (supervision by Prof. M. Elad and Prof. Y. C. Eldar, 2009), and PhD (supervision by Prof. M. Elad 2014) degrees from the Department of Computer Science, The Technion - Israel Institute of Technology, Haifa. Raja was a postdoc at the computer science department at the Technion (Nov. 2013 till July 2014) and at the lab of Prof. G. Sapiro at Duke University, Durham, USA (July 2014 and Aug. 2015). His research interests lie at the intersection between signal and image processing and machine learning, and in particular, in deep learning, inverse problems, sparse representations, and signal and image modeling.
Raja received the Maof prize for excellent young faculty (2016-2019), VATAT scholarship for excellent postdoctoral fellows (2014-2015), Intel Research and Excellence Award (2005, 2013), the Excellence in Signal Processing Award (ESPA) from Texas Instruments (2008) and was part of the Azrieli Fellows program (2010-2013). He has co-organized workshops and tutorials on deep learning in leading conference such as ICML 2016, ICCV 2015, CVPR 2016, EUSIPCO 2016 and ACCV 2016.
How structure can improve the theory and practice in neural networks?
Abstract:
The past five years have seen a dramatic increase in the performance of recognition systems due to the introduction of deep architectures for feature learning and classification. However, the mathematical reasons for this success remain elusive. In this talk we will briefly survey some existing theory of deep learning. In particular, we will focus on data structure based theory and discuss two recent developments.
We first study the generalization error of deep neural network. We will show how the generalization error of deep networks can be bounded via their classification margin. We will also discuss the implications of our results for the regularization of the networks. For example, the popular weight decay regularization guarantees the margin preservation, but it leads to a loose bound to the classification margin. We show that a better regularization strategy can be obtained by directly controlling the properties of the network’s Jacobian matrix.
Then we focuse on solving minimization problems with neural networks. Relying on recent recovery techniques developed for settings in which the desired signal belongs to some low-dimensional set, we show that using a coarse estimate of this set leads to faster convergence of certain iterative algorithms with an error related to the accuracy of the set approximation. Our theory ties to recent advances in sparse recovery, compressed sensing and deep learning. In particular, it provides an explanation for the successful approximation of the ISTA (iterative shrinkage and thresholding algorithm) solution by neural networks with layers representing iterations.
​
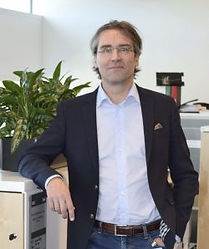
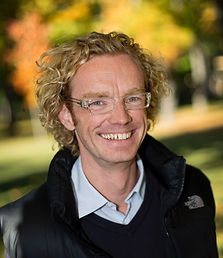
Prof. Henrik Boström
Stockholm University & RISE SICS
Short Bio: Henrik Boström is professor at the Dept. of Computer and System Sciences at Stockholm University and senior researcher at RISE SICS. His research interest is primarily within machine learning with a particular focus on ensemble learning, interpretable models, including decision trees and rules, and conformal prediction. He is action editor of the Machine Learning journal and on the editorial boards of Data Mining and Knowledge Discovery, Journal of Machine Learning Research and Intelligent Data Analysis. He is a frequent area chair and program committee member of several of the most prominent conferences in the area.
Conformal Prediction
Abstract:
Conformal prediction (CP) has recently been gaining increased attention as a framework for quantifying the uncertainty of predictions. When employing the framework, which can be used with any standard learning algorithm, the probability of making incorrect predictions is bounded by a user-provided confidence threshold. In this talk, we will briefly introduce the framework and illustrate its use in conjunction with both interpretable models, such as decision trees, and highly predictive models, such as random forests.
​
​
​
​
​
​
Prof. Thomas Schön
Uppsala University
Department of Information Technology,
Division of Systems and Control,
751 05 Uppsala, Sweden.
Short Bio: Thomas B. Schön is Professor of the Chair of Automatic Control in the Department of Information Technology at Uppsala University. He received the PhD degree in Automatic Control in Feb. 2006, the MSc degree in Applied Physics and Electrical Engineering in Sep. 2001, the BSc degree in Business Administration and Economics in Jan. 2001, all from Linköping University. He has held visiting positions with the University of Cambridge (UK), the University of Newcastle (Australia) and Universidad Técnica Federico Santa María (Valparaíso, Chile). He received the Tage Elander prize for natural sciences and technology in 2017 and the Arnberg prize in 2016, both awarded by the Royal Swedish Academy of Sciences (KVA). He was awarded the Automatica Best Paper Prize in 2014, and in 2013 he received the best PhD thesis award by The European Association for Signal Processing. He received the best teacher award at the Institute of Technology, Linköping University in 2009. He is a Senior member of the IEEE and an Associate Editor of Automatica. More information about his research can be found on his website: user.it.uu.se/~thosc112
Learning flexible models of nonlinear dynamical systems
​
Abstract: A key lesson from modern machine learning is that flexible models often give the best performance. The two standard ways of building flexible models are: i) we make use of a large but fixed number of parameters or ii) we let the number of parameters grow with the size of the dataset (often referred to as a nonparametric model). An popular example of the first is deep learning and the Gaussian process constitutes a particular example of the latter. When it comes to dynamical systems, the nonlinear state space model is a rich and useful representation. I will in this talk show how we can build a nonparametric nonlinear state space model inspired by the Gaussian process. This results in a flexible probabilistic representation capable of capturing and explaining nonlinear dynamics in a seemingly useful fashion. We learn the model using a tailored particle filtering algorithm. If time permits I will towards the end of the talk also show some of our related ongoing research.
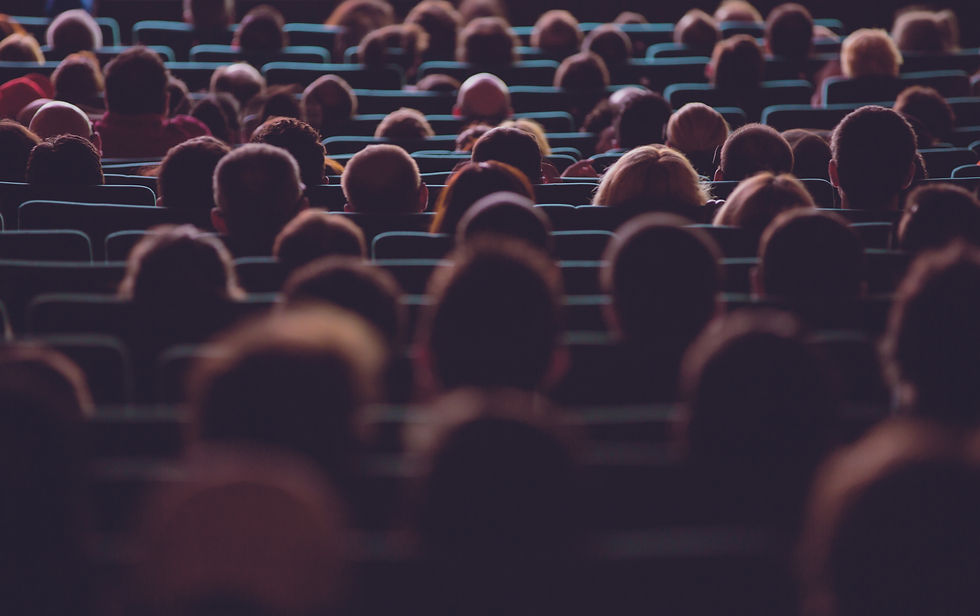
ACCESS - Autonomic Complex Communication nEtworks, Signals and Systems - has enabled KTH to bring together 160 researchers from Electrical Engineering, Computer Science, and Mathematics in order to carry out long-term interdisciplinary research and joint PhD education on complex networked communication systems.
It is a networked world. People communicate independent of distance and time; systems communicate with systems as connectivity becomes a natural feature of any electronic device. Networked systems are vital infrastructures in our society and the basis of more and more services that we take for granted; from connectivity of personal devices in the home and car to global communication and positioning via satellite systems.
At the core of these systems are some fundamental technical issues: they must be designed to carry out the intended functions; the systems must perform efficiently and predictably; they must be manageable, controllable, and upgradeable and they must be reliable to the point of being infallible. Together with industrial partners, ACCESS researchers at KTH aim at developing fundamental understanding and engineering principles for designing self-managed and scalable communication networks in which applications may share real-time information and cooperate in an efficient, affordable, and reliable manner.
​
Adaptive Sketching and Validation for Learning from Big Data
Abstract:
We live in an era of data deluge. Pervasive sensors collect massive amounts of information on every bit of our lives, churning out enormous streams of raw data in various formats. Mining information from unprecedented volumes of data promises to limit the spread of epidemics and diseases, identify trends in financial markets, learn the dynamics of emergent social-computational systems, and also protect critical infrastructure including the smart grid and the Internet’s backbone network. While Big Data can be definitely perceived as a big blessing, big challenges also arise with large-scale datasets. This talk will put forth novel algorithms and present analysis of their performance in extracting computationally affordable yet informative subsets of massive datasets. Extraction will effected through innovative tools, namely adaptive censoring, random subset sampling (a.k.a. sketching), and validation. The impact of these tools will be demonstrated in machine learning tasks as fundamental as (non)linear regression, classification, and clustering of high-dimensional, large-scale, and dynamic datasets.